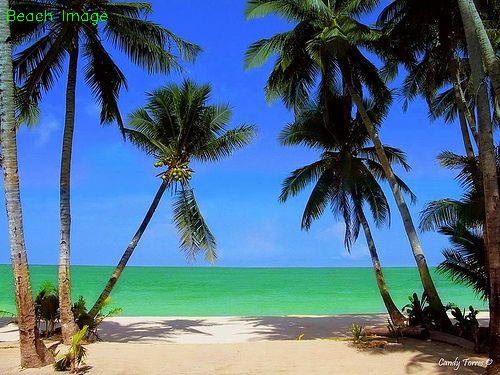
Beach classifier, I wish I was here.
I have been burning the midnight oil finishing up a project for my Computational Photography course at Columbia University. For this project we had to make two classification systems, one which classified beach and grassland imagery using a given feature vector description, and a second classifier for any two objects using whatever technique we wished to generate the feature vectors. It was suggested that we do our work in Matlab, but we had the option to work in C++. I opted for the latter as I really wanted to write something that I could possible re-use in another project. The final system was developed under Windows using Visual Studio 9, and makes liberal use of OpenCV 2.2 and LibSVM 3.0.
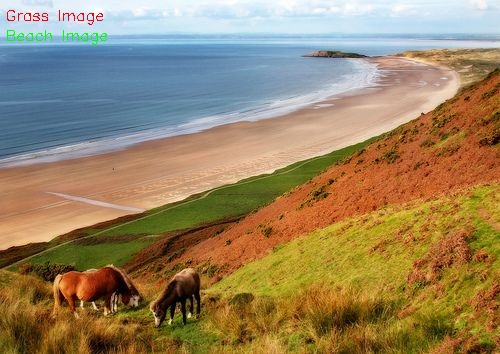
"Misclassification"
The beach / grassland images were classified by dividing the image into a three by three grid and calculating the color average, color standard deviation, and color skew for each of the HSV channels. This feature vector was then used in a support vector machine with a linear kernel. The overall error rate was 13.33%. For the beach images 11.67% were misclassified as grassland, while 15.00% of the grass images were classified as beach. The classification is written in the top, left corner. If the image was misclassified there are two values listed. The red value is the classification, and the green value is true value.
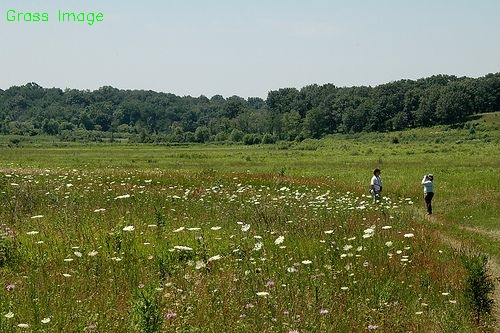
Correctly Classified Grassland
For the second part of the project I wrote a system that classifies images as either screws or nails. The system assumes that both of these objects are aligned to be roughly vertical. I wrote a separate class awhile back that would re-orientate the images based on the major axis of the extracted contour. To do the classification I first thresholded the gray scale image and then extracted the resulting contour. After doing this a few morphological operations were performed on the contour and the Hu-moments and a few other statistics were calculated. I also applied the Canny edge detector to the images and piped the results into a Hough line detector. The results of the line detector were then binned according to length and orientation. This data was used to generate a feature vector which was used for classification via a support vector machine with a linear kernel. The overall error rate was 3.58%. 1.33% of the screw images were misclassified as nails, while 7.27% of the nail images were misclassified as screws. The classification is written in the top, left corner. I used the letter “N” to indicate nails, and the letter “S” to indicate If the image was misclassified there are two values listed. Some results are shown below.
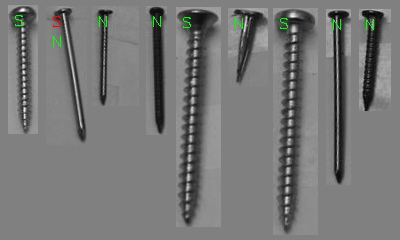
Samples from the screw / nail classifier
The complete set of beaches and grassland images can be found in my
beach / grassland classification set on flickr along with the complete set of
screw/nail classification results. The code is posted on my
computational photography Google code page. The code was written under the gun so it isn’t nearly as clean as I would like it to be, and everything is very data set specific. Hopefully once the semester is over I can go back and refactor it to be a more general solution.